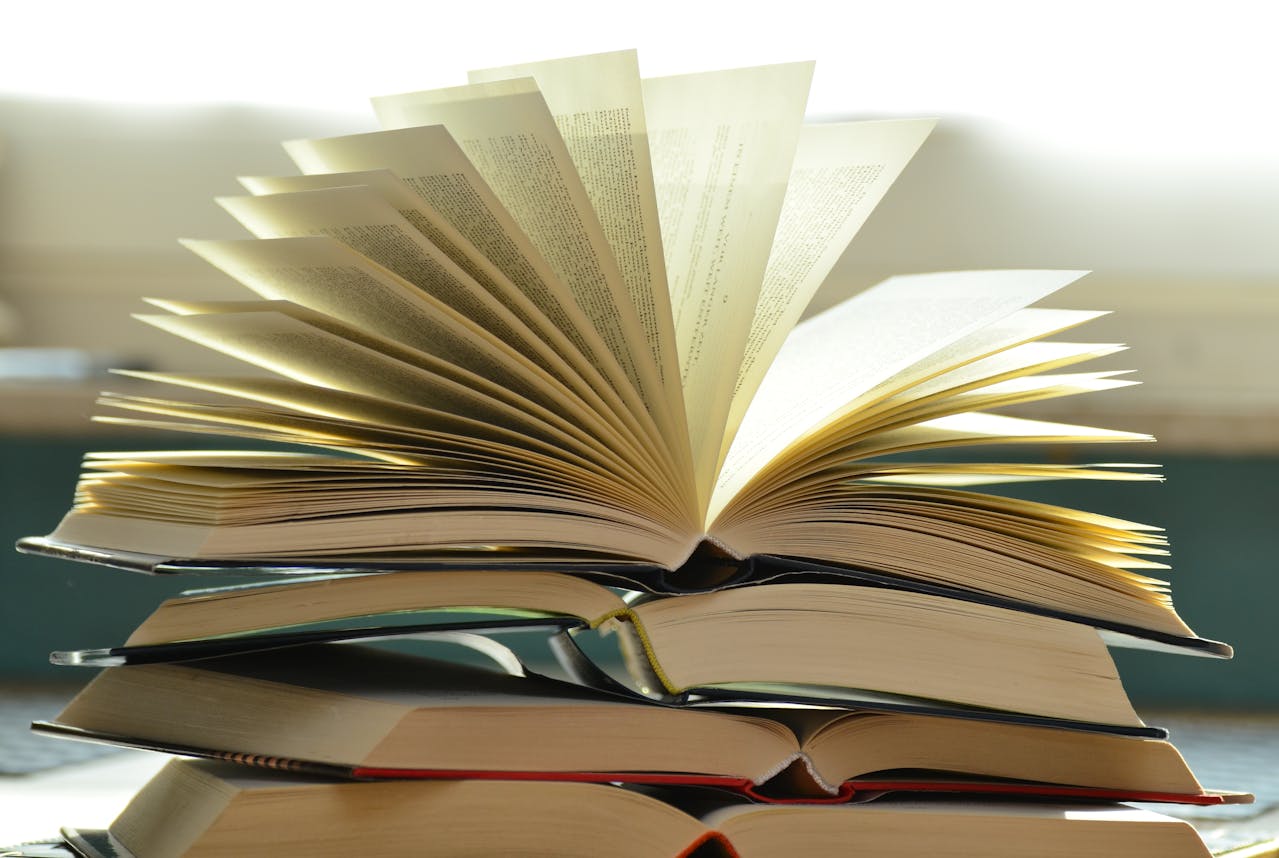
AI Analysis of Large Qual Datasets: How Big is Too Big for Reliable Qualitative Insights?
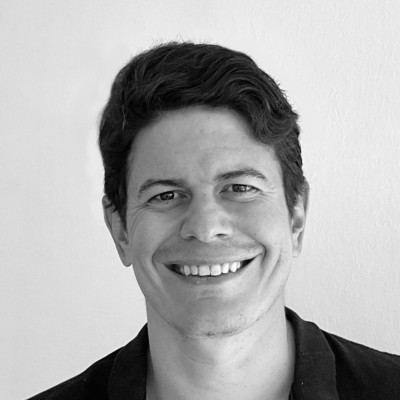
Introduction: The Allure of the Large Context Window
In our last post, "Expanding Horizons: Large Context Windows and Enhanced AI Capabilities in Research", we explored the massive expansion of context windows in Large Language Models (LLMs). The ability to potentially feed dozens of interview transcripts or extensive online community data into an AI for analysis at once sounds like a game-changer for qualitative research. It promises coherent analysis across vast amounts of data, something previously impossible, but what are the practical limits of that promise?
How Big is Too Big? When Capacity Outstrips Reliability
While the capacity is impressive, simply having a larger container doesn't guarantee the quality of what comes out. As we feed more and more data into these large context windows, a question arises: how much is too much before the AI starts to struggle?
Think of it like trying to synthesize the key themes from hundreds of documents spread out simultaneously on a giant table – while you can see everything at once, accurately picking out and connecting specific details becomes incredibly difficult. Similarly, LLMs, despite their power, can face challenges recalling and synthesizing information when overwhelmed. This is often highlighted by "Needle in a Haystack" tests, which show that an AI's ability to find specific facts ("needles") can decrease as the volume of surrounding text ("haystack") grows, especially if the information is buried deep within the context (the "lost in the middle" problem). Indeed, even the creators of leading models like ChatGPT acknowledge these recall challenges in their own documentation.
Key Factors to Consider Before Going "All In"
So, when deciding how much data to analyze at once using AI, you need to weigh a couple of critical factors:
-
The Sheer Size of Your Dataset:
- What it means:
-
Simply, how many pages of transcripts, posts, or documents are you trying to analyze simultaneously?
- The Catch:
-
While a model might boast a 1 million token context window (potentially holding ~70-80 interview transcripts), asking it to analyze all of that coherently at once pushes its capabilities to the limit.
(Note: These capability estimates reflect models available as of April 2025 and are subject to change as AI technology evolves.)
- Example:
-
Analyzing 5 related interview transcripts is likely manageable. Trying to synthesize nuanced themes across 75 transcripts significantly increases the risk of the AI missing connections or misinterpreting details buried in the middle. The potential is there, but the reliability might decrease.
-
The "Needles" You're Looking For (Task Complexity):
- What it means:
-
What are you actually asking the AI to do with the data? Are you looking for a single, simple piece of information, or are you asking for complex synthesis across multiple points?
- The Catch:
-
Finding one specific "needle" is much easier for an AI than finding and weaving together multiple "needles" scattered throughout the haystack.
- Example 1 (Simpler Task - Fewer, Clearer Needles):
-
Asking the AI to "Extract all direct quotes mentioning dissatisfaction with Product Feature X" across 10 transcripts might yield reliable results even in a reasonably large context. The task is specific and involves finding distinct items.
- Example 2 (Complex Task - Multiple, Interconnected Needles):
-
Asking the AI to "Analyze the evolution of sentiment towards our service recovery process across 50 interviews, identifying the key turning points and how early complaints relate to later expressions of satisfaction" is far more complex. This requires the AI to track multiple subtle shifts, compare points made far apart, and synthesize a nuanced narrative – tasks where reliability can significantly degrade in very large contexts.
Bridging the Gap: Evaluating Your Analysis Task
Understanding these limitations is crucial, but how do you apply this knowledge in practice? Simply guessing isn't sufficient. Before you run a complex analysis across a large dataset, you should evaluate your intended query considering factors like:
- The volume of data you've selected.
- The type of analytical task you're asking the AI to perform (e.g., simple extraction vs. complex synthesis).
- Known patterns of AI reliability based on AI model, context size and task complexity.
Making this pre-analysis assessment accurately is not always straightforward without dedicated assistance – as I'll detail later regarding a specific solution within the IO Platform. However, evaluating these factors before analysis is essential. It’s the critical step that separates harnessing AI's power effectively from simply generating large volumes of potentially unreliable text, allowing us to move towards achieving truly meaningful and trustworthy insights.
Conclusion: Harnessing Power with Awareness
The expansion of large context windows represents an important advancement in the AI capabilities available to qualitative researchers, offering unprecedented scale. However, maximizing their value requires understanding their limitations. By considering your dataset size and the complexity of your analytical task – and ideally, using tools designed to help evaluate these factors – you can approach large-scale AI analysis with greater confidence and achieve more reliable, meaningful insights.
Putting Theory into Practice: The IO Platform Solution
As mentioned earlier, the IO Platform offers an integrated tool designed to provide guidance with the challenge of assessing the potential reliability of complex AI analysis tasks based on your selected data size, chosen AI model, and specific query type. It helps researchers navigate the trade-offs before committing to a specific analysis approach.
- It might confirm that your analysis request is well-suited for the selected data size.
- Or, it might flag potential reliability concerns, suggesting that the task is very complex for the amount of data provided in a single context.
- In such cases, it might recommend alternative strategies, such as breaking the analysis into smaller, more manageable chunks (using the chunking techniques we also support) or refining the query to be more focused.
This aims to empower researchers, helping you leverage the power of large context windows more effectively while being aware of, and mitigating, the potential pitfalls. It’s about leveraging AI effectively by understanding and working within its limits.
Related Posts
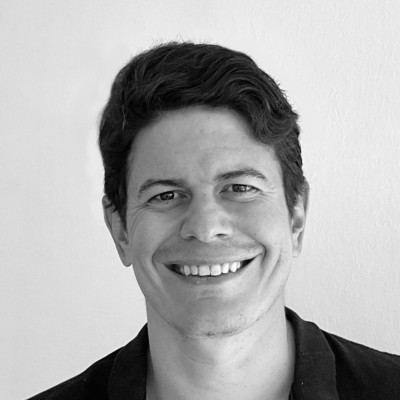
Néstor Fernández Conde
Founder and lead developer at InOpinia with a focus on creating intelligent, integrated platforms for modern qualitative research. PhD in astrophysics with 15+ years in research technology.
Connect on LinkedInInterested in learning more about the IO platform and how it can enhance your qualitative research?